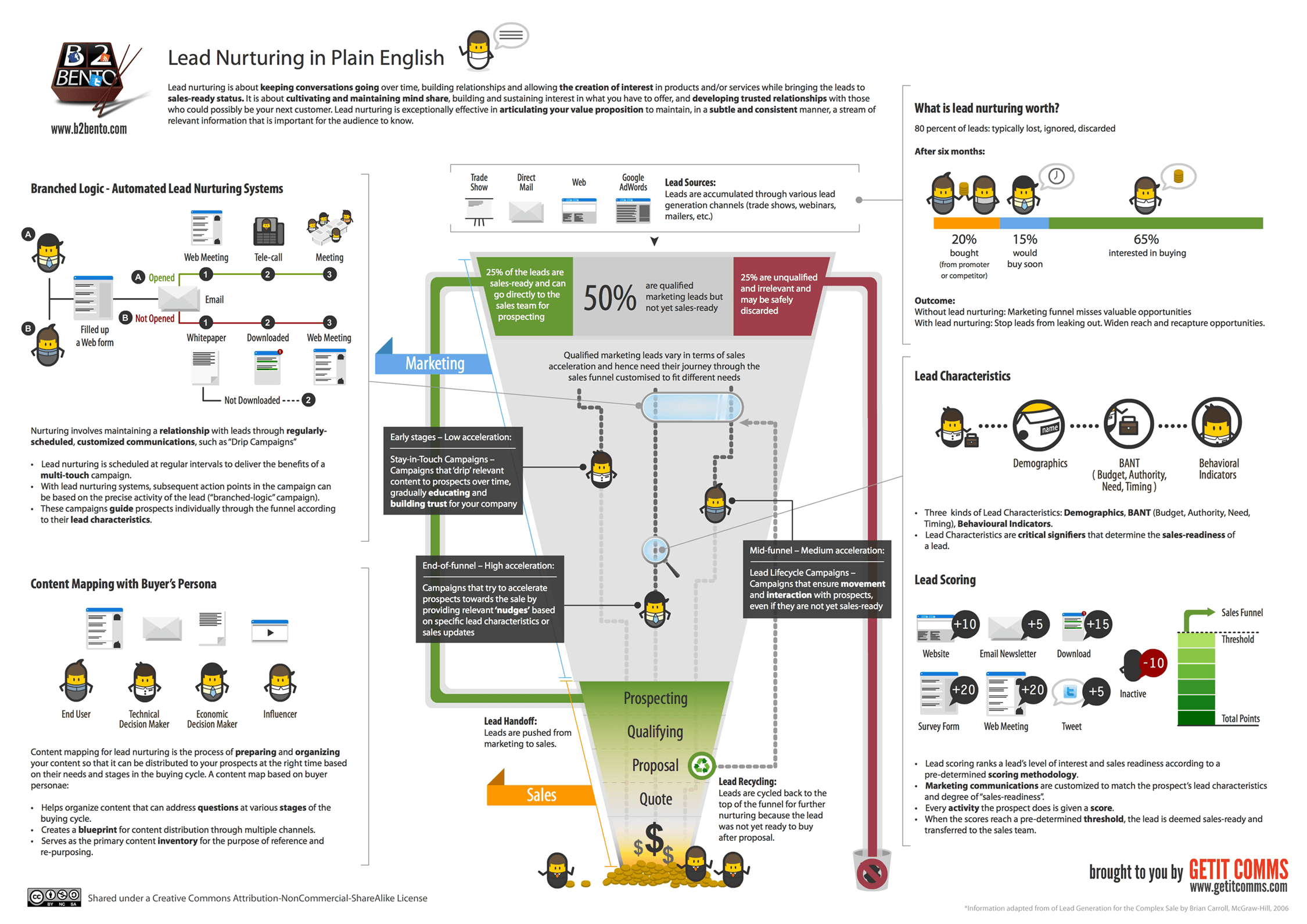
Scoring a lead comes with a massive amount of questions that at times can feel endless. At what point does the marketing hand over the MQL (Marketing Qualified Leads) to your sales team? At what point does your MQL become SQL (Sales Qualified Lead)? How do you score such MQLs who have shown some level of interest in the services offered by your business? How many MQLs do you need in your pipeline to support sales and increase revenue? What should be the lead score threshold for MQLs? This is the conundrum often faced by marketing and sales leaders in B2B organizations when it comes to defining what actually makes a good lead.
Know this – there isn’t a universal standard or a one-size fits all model.
We have asked various sales leaders for their opinion on this topic and here is the essence of what they said, from defining the problem to giving the solution.
Why do you need lead scoring?
The necessity for setting up effective lead scoring sprouts from the fact that the sales funnels need a certain number of high quality leads for conversions to happen. The long-term purpose of lead scoring is to identify where your prospects are in the sales cycle and predict their readiness to buy from your sales reps.
Lead scoring acts as the predictability engine, improves forecast accuracy, improves conversion rate and helps you plan your sales accordingly.
Some Lead Scoring Mistakes
Setting up lead score threshold can be a daunting task and it varies from businesses to businesses. There is one major mistake that most marketers in the B2B selling space make, which is compromising quality over quantity. They pass on the leads to the SDRs at a very early stage with the anticipation that “any lead” will convert. In such cases, the threshold is very low, and lead nurturing with relevant information might not have happened on the way. Low or no threshold drives to low conversion rates and wastage of organization’s resources.
This often stems from another mistake further upstream, which is not refining the lead score model or in some cases, not using one at all. Without this system in place, it quickly becomes a free-for-all with cascading problems downstream.
Watch out, if your business is making these mistakes. Study the data to see the correlation between MQLs and Opportunities created as well as closed.
Refine Lead Score Model and Threshold for MQLs
Oftentimes, businesses forget that lead scores must be continuously monitored and tweaked. At the center of it is various data points, which need to be referenced both by your sales and marketing teams. The following practices will help you refine your lead score:
- Sometimes you might notice that the quite a few leads with low scores get converted to MQL too. Does that mean you lead scoring is plain wrong? No, it means you need to study the leads to find what was common between all the leads that converted irrespective of their scores.
- Follow the above step with the leads that converted from MQL into SQL, and so on and so forth all the way through to close. A free resource to view deal closures by lead source can be found here
- Similarly, study the lost leads to find the points common between them, especially the ones with high lead scores.
- Based on these data points tweak your scoring system and assign relevant weights to these attributes.
- To have a better scoring threshold and a higher correlation between score and won deals, revise the scores once in every few weeks.
Methods to Analyze Your Current Lead Score Threshold
Start with the Statistical Analysis of your current model by scrutinizing the historical data based on your sales cycle:
- What is the score of opportunities that were successfully won?
- Which score scale gives a higher number of opportunities?
- What is the conversion rate at each score range after they are handed over to the SDRs?
- What change is applied to the score after each successful conversion?
- What percentage of MQLs turn into SQLs? A minuscule percentage implies your marketing is not passing highly qualified leads to the sales team.
- What percentage of low scoring leads or false negatives end up converting? If that percentage is high it implies you haven’t set the lead scoring right. Study such leads closely to find the missing rules.
Next, is Qualitative Analysis to find the following:
- What percentage of leads end up on the list of false positives vs false negatives. False positive is the list of MQLs that get abandoned by the sales team. False negatives are the low score but converted leads. Get an insight on what leads to such false positives and false negatives, what the ratio between them is, and how that ratio compares to your overall win-rate.
- Sometimes you have false positives because of the wrong criteria for scoring the leads (more on it in the section below).
Fix Lead Score Threshold for MQLs
Generally, B2B businesses build their lead scoring model on demographic and behavioral criteria. Demography of the target has to match their ideal customer persona. Behavioral criteria are tracking the way the lead is interacting with various sales and marketing personnel/resources. The lead score threshold is plotted based on either, or both, of these two criteria.
As an example, let’s say your email campaign has reached the inbox VP of Sales of your target customer. They fit into your buyer’s profile being one of the major decision-makers on the buyer’s side but apart from opening the email, they might not have engaged with any of your content/ landing pages/ websites for months. If leads such as this get a high score and lands above your MQL score threshold, they will automatically get routed to your sales team and bring no conversion but instead just waste their time, energy, and focus!
Here are some fixes for this situation:
Use NxM grid to map demographic elements against the behavioral aspects:
Sort and label every element of demographic criteria as A/B/C/D/F on one axis and on another axis plot all the latent plus active behaviors as numbers from 1….n. Based on this you will get an alphanumeric ID perspective which will give you a clear understanding of where your MQL threshold should be.
Use Binary Logistic Regression (BLR):
This one is a completely scientific method. With this, you get a very strong probability on whether the lead will convert or not. This is regarded as one of the most sound techniques to determine the ideal score threshold and predict either qualified opportunity or non-qualified opportunity based on all the independent-dependent variables (in the form of demographic and behavioral criteria). We won’t dip into the details of it in this article, but it’s worth a quick google search if you have a strongly data-centered process.
One final thought
Last but not the least, throughout this process of identifying your ideal score threshold, your marketing should be communicating and constantly discussing with the sales team.
No one knows the pulse of buyers better than your sales team. They have the best insight on where your scoring model is breaking down.
Has your business nailed the threshold for MQLs using one of these techniques? What about something not mentioned here? We’d love to hear your experience and thoughts on this! If you find this interesting, be sure to check out what we offer in the sales forecasting section here, where we leverage AI to handle things like this completely hands-off.